Artificial intelligence (AI) is rapidly transforming our world, from facial recognition software unlocking smartphones to chatbots handling customer service inquiries. But behind the magic of AI lies a crucial, often unseen process: data annotation. This meticulous process involves labeling data ā images, videos, text, or audio ā with meaningful information to train AI models to recognize patterns, objects, and concepts.
In essence, data annotation acts as the bridge between the raw, unprocessed data and the intelligent capabilities of AI models. Let’s delve deeper into this fascinating field, exploring its workings, applications, and the future of this essential aspect of AI development.
How Does Data Annotation Work?
Data annotation involves a series of steps, typically performed by human annotators:
-
Data Selection and Preprocessing: The data used for annotation must be carefully chosen to represent the real-world scenarios the AI model will encounter. Preprocessing might involve cleaning the data, removing irrelevant information, or standardizing formats.
-
Task Definition and Annotation Guidelines: Clear instructions outlining the specific information to be labeled are crucial. For example, in image annotation for self-driving cars, guidelines might specify labeling traffic signs, pedestrians, and lane markings.
-
Annotation Tools: Specialized software platforms streamline the annotation process. These tools allow annotators to easily label data points, draw bounding boxes around objects, or transcribe text.
-
Quality Assurance: To ensure the accuracy of annotated data, a robust quality assurance process is essential. This might involve double-checking annotations or using automated tools to identify inconsistencies.
Types of Data Annotation:
Data annotation can be categorized based on the type of data being labeled:
-
- Image Annotation: This involves labeling objects, landmarks, or scenes within images. Common tasks include image classification (identifying the content of an image), object detection (pinpointing specific objects within an image), and image segmentation (assigning labels to each pixel in an image).

-
- Video Annotation: Similar to image annotation, video annotation involves labeling objects, actions, or events within video footage. This might involve tracking the movement of objects, identifying activities, or transcribing spoken words.
-
- Text Annotation: This involves labeling text data with additional information, such as sentiment analysis (identifying positive, negative, or neutral sentiment in text), topic modeling (identifying the main themes of a text), or named entity recognition (identifying people, organizations, or locations mentioned in text).
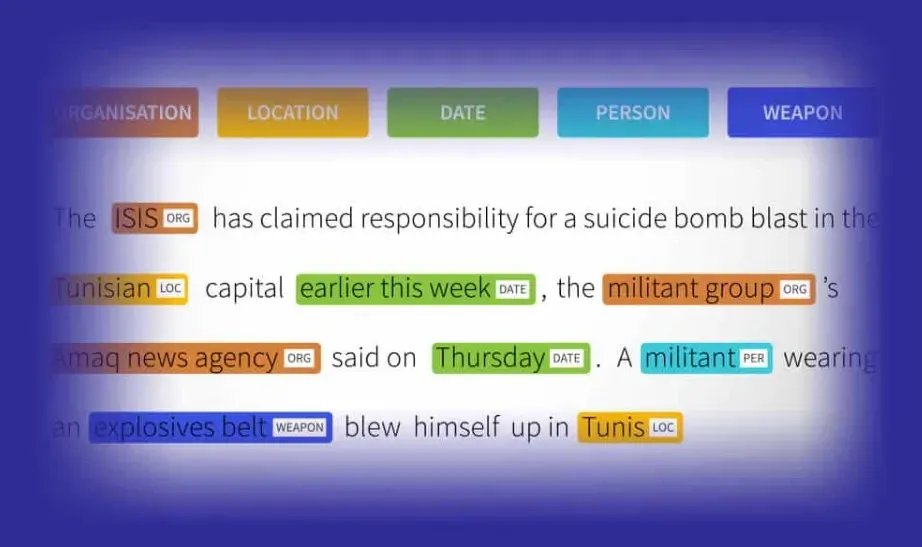
-
- Speech Annotation: Speech annotation involves transcribing spoken words into text or labeling emotions and sentiment conveyed in speech data.
Applications of Data Annotation
Data annotation underpins a vast array of AI applications across various industries:
-
Self-driving Cars: Annotated images and videos train AI models to recognize objects like pedestrians, traffic signs, and lane markings, enabling safe autonomous navigation.
-
Facial Recognition: Annotated images help AI models accurately identify individuals, paving the way for security applications and personalized experiences.
-
Medical Diagnosis: Annotated medical images assist AI models in detecting diseases or abnormalities in X-rays, CT scans, and other medical imaging data.
-
Natural Language Processing (NLP): Annotated text data empowers AI models to understand the nuances of human language, enabling applications like chatbots, machine translation, and sentiment analysis.
-
Computer Vision: Annotated images and videos train AI models to interpret visual information, leading to advancements in object recognition, image search, and robotics.
The Future of Data Annotation
As AI technology continues to evolve, so too will the field of data annotation. Here are some key trends to watch:
-
Automation: While human expertise remains crucial, advancements in active learning and semi-supervised learning techniques will automate certain aspects of data annotation, increasing efficiency.
-
Crowd-sourcing: Platforms leveraging a global workforce of annotators can provide cost-effective solutions for large-scale data annotation projects.
-
Focus on Quality: With the growing importance of responsible AI, ensuring unbiased and high-quality annotated data will become a top priority.
-
Domain-specific Tools: Specialized tools tailored to specific industries (e.g., healthcare, finance) will streamline the annotation process and improve accuracy.
-
Privacy and Security: As data privacy regulations become more stringent, robust data security measures will be essential for protecting sensitive information during annotation.
Data Annotation FAQ
What are the skills required to be a data annotator?
Data annotation requires strong attention to detail, good visual acuity for image and video annotation, and basic computer literacy. Familiarity with the specific domain being annotated (e.g., medical terminology for healthcare data) can be beneficial
How much do data annotators earn?
Data annotator salaries vary depending on experience, location, and the complexity of the project. Entry-level positions might start at minimum wage, while experienced annotators with specialized skills can earn significantly more.
Is data annotation a good career choice?
Data annotation can be a good career choice for individuals with strong attention to detail and a keen interest in AI technology. The field offers flexible work arrangements and opportunities to contribute to the development of cutting-edge AI applications. However, it can also be repetitive work, and career advancement might require acquiring additional skills in project management or data science.
What are the ethical considerations in data annotation?
Data annotation raises ethical concerns, particularly regarding bias and data privacy. Annotator biases can inadvertently be reflected in the labeled data, impacting the fairness and accuracy of AI models. Additionally, ensuring the security and privacy of sensitive data during annotation is crucial.
How can I get started with data annotation?
Several online platforms offer data annotation jobs. Look for reputable companies with clear quality standards and fair compensation practices. Consider starting with entry-level projects to gain experience and build your portfolio. There are also online courses and tutorials available to learn best practices in data annotation.
What are the future prospects for the data annotation industry?
The demand for data annotation is expected to grow significantly as AI development continues to accelerate. Advancements in automation will likely reshape the field, with a focus on human expertise for complex tasks and quality control. Staying updated on the latest trends and acquiring additional skills can help individuals thrive in the evolving world of data annotation.
Is there anything else I can do besides data annotation to contribute to AI development?
Absolutely! While data annotation plays a crucial role, there are other avenues for contributing to AI. Here are a few examples:
-
Data Science: Data scientists are responsible for collecting, analyzing, and preparing data for AI models. This field requires expertise in statistics, programming, and machine learning.
-
Machine Learning Engineering: Machine learning engineers design, build, and deploy AI models. They possess skills in software development, cloud computing, and machine learning algorithms.
-
AI Research: Researchers are at the forefront of developing new AI algorithms and techniques. This field requires a strong foundation in computer science, mathematics, and deep learning concepts.
-
AI Ethics: As AI becomes more integrated into society, ensuring its ethical development becomes critical. Professionals in this field advocate for responsible AI development and address issues like bias and fairness in AI systems.
The field of AI development is vast and offers diverse opportunities for individuals with varying skillsets and interests. Data annotation serves as a valuable entry point, and the knowledge gained can be a stepping stone to further exploration within the exciting field of AI.
-
PreciousĀ Gabraels (Precious Okechukwu Nwosu) is a tech enthusiast. A professional Web Developer, Blogger, SEO Specialist, and Digital Marketer. He is also a co-founder of Nova Vamp Web Design Consultancy which specializes in the digitization of businesses.
View all posts
Tags: AI annotation data annotation